Unlocking the Power of Image Datasets for Object Detection
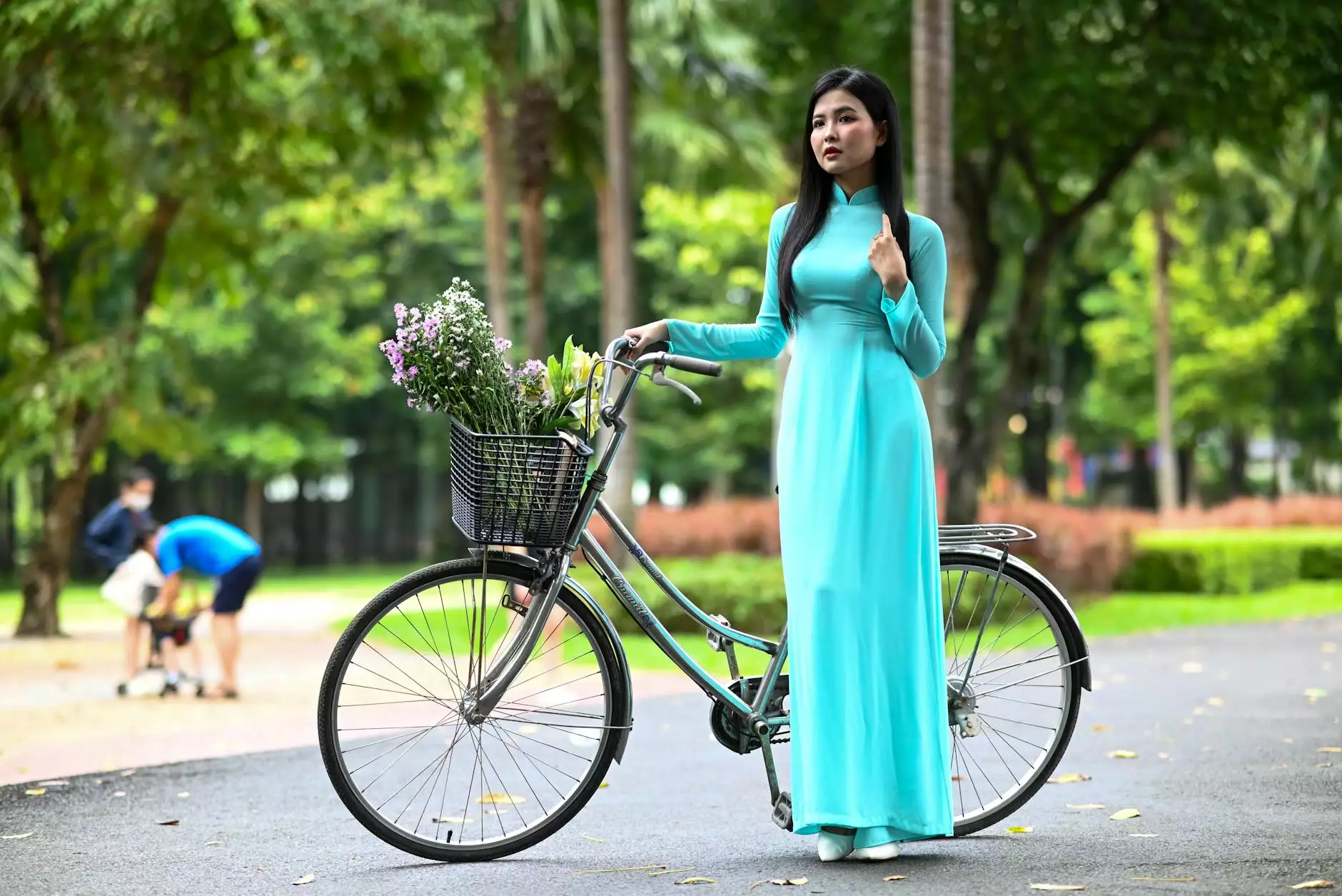
In today's technology-driven world, the demand for precise object detection systems has skyrocketed. The ability of machines to interpret visual data is crucial for sectors ranging from autonomous vehicles to medical imaging. Central to the success of these systems is the image dataset for object detection. In this article, we delve deep into what image datasets are, their relevance to object detection, and how leveraging the right data annotation tools can elevate your outcomes.
The Fundamentals of Image Datasets
An image dataset consists of a collection of images ideally labeled for specific purposes, notably for training machine learning models. The effectiveness of an object detection system heavily relies on the quality and diversity of its dataset. Here are some key characteristics:
- Diversity: A wide array of images covering various scenarios, angles, and lighting conditions help the model generalize better.
- Quality: High-resolution images with clear labeling make the annotation process smoother and facilitate better model training.
- Annotations: Accurate and detailed annotations (bounding boxes, segmentation masks, etc.) are essential for teaching models to recognize and classify objects correctly.
Why Are Image Datasets Crucial for Object Detection?
The importance of image datasets in the realm of object detection cannot be overstated. Here are some reasons why:
- Model Accuracy: The more comprehensive the dataset, the higher the chances that the model will perform accurately in real-world applications.
- Generalization: Datasets that include a wide variety of examples help models learn to identify objects across different contexts, reducing bias.
- Scalability: Having a structured dataset allows businesses to scale their machine learning efforts efficiently across multiple projects.
Building a Robust Image Dataset for Object Detection
Creating an effective image dataset for object detection involves several steps:
1. Collecting Images
Start by gathering images that represent the objects you want to detect. This process may include:
- Using stock photo platforms.
- Taking custom photographs in controlled conditions.
- Web scraping for publicly available images (while respecting usage rights).
2. Annotation Process
Once you have your images, the next step is annotation. Effective annotation can significantly impact your model's performance. You can either annotate the images manually or use automated solutions provided by advanced data annotation platforms like Keylabs.ai. Manual annotation often requires:
- Identifying the objects within each image.
- Creating bounding boxes around detected objects.
- Labeling each bounding box accurately.
3. Validation
Having a second pair of eyes review your dataset is crucial. This validation step ensures that:
- The annotations are correct, reducing potential data errors.
- All images in the dataset are useful and meet quality standards.
4. Regular Updates
Technology evolves, and so should your datasets. Regularly updating your image dataset for object detection can help in accommodating new object types, improving classification accuracy, and staying relevant in your field.
Keylabs.ai: Your Partner in Data Annotation
At Keylabs.ai, we provide high-end data annotation tools designed to simplify and enhance your workflow when building image datasets for object detection. Our platform offers:
Advanced Annotation Tools
Utilize our cutting-edge tools to annotate images swiftly and accurately. Our intuitive interface allows for seamless annotation, making it accessible even for those new to this technology.
Scalability and Flexibility
Whether you're a small startup or a large enterprise, our platform scales according to your needs. You can annotate thousands of images without sacrificing quality or speed.
Expert Support
Our team of experts is always available to assist you, ensuring that you get the most out of our platform.
Real-World Applications of Object Detection
The applications of object detection using robust image datasets are vast and varied. Here are some notable industries that benefit:
1. Autonomous Vehicles
Using object detection capabilities, self-driving cars can identify obstacles, pedestrians, and road signs, making our roads safer.
2. Retail and Inventory Management
Object detection can streamline inventory processes, enhance customer experience through automated checkout systems, and analyze shopper behavior.
3. Healthcare
In the medical sector, object detection can aid in diagnosing diseases by analyzing medical images, leading to quicker and more accurate treatment options.
4. Security and Surveillance
Enhanced security systems use object detection to identify unusual activities and alert security personnel in real-time.
Best Practices for Working with Image Datasets
To maximize the performance of your object detection models, consider these best practices:
1. Ensure High-Quality Images
High-resolution images yield better results. Avoid blurry or poorly lit images that might confuse the model during training.
2. Use a Balanced Dataset
A balanced dataset ensures that all classes are equally represented, which is crucial for training a fair and unbiased model.
3. Incorporate Data Augmentation
Utilize data augmentation techniques to artificially expand your dataset, improving model robustness against various scenarios.
4. Leverage Transfer Learning
Using pre-trained models with your datasets can significantly reduce training time and enhance accuracy from the get-go.
Conclusion: The Future of Object Detection
In conclusion, the role of an image dataset for object detection is pivotal in the age of AI and machine learning. As industries continue to adopt these technologies, the demand for precise, well-annotated datasets will only increase. Partnering with tools like those offered by Keylabs.ai can propel your business forward, allowing you to leverage these innovations effectively. Make your move today, enhance your data capabilities, and pave the way for a smarter future.