The Importance of Annotated Image Datasets in Modern AI
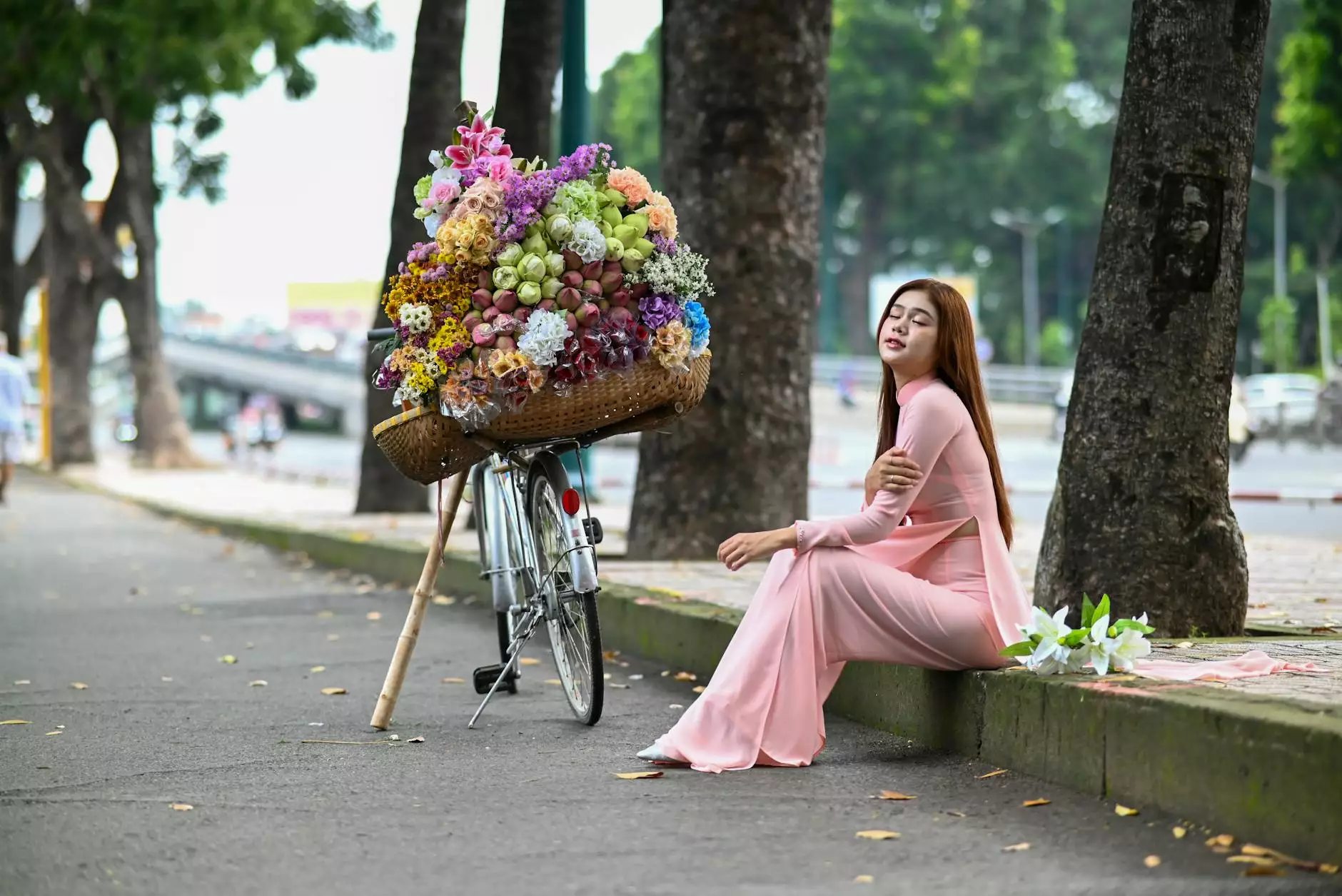
In the rapidly evolving world of technology, the role of artificial intelligence (AI) is becoming increasingly significant. One of the building blocks of effective AI systems is the quality of data on which they are trained. In this context, annotated image datasets emerge as a vital component, playing a pivotal role in how machines learn and make decisions. This article offers a comprehensive insight into the significance of annotated image datasets, particularly through the lens of data annotation tools and platforms, prominently featuring keylabs.ai.
Understanding Annotated Image Datasets
To grasp the impact of annotated image datasets, we must first define what they are. An annotated image dataset is a collection of images that are accompanied by labels or annotations that describe the contents of the images. These annotations can vary widely, encompassing a variety of formats, including:
- Bounding Boxes: Used for object detection tasks, highlighting the location of objects within the image.
- Segmentation Masks: Providing pixel-level annotations to define the exact shape of objects in the image.
- Keypoints: Marking specific points on objects, often used in tasks like pose estimation.
- Text Annotations: Describing the scene or context beyond just the visual content.
Why Annotated Image Datasets Are Essential for AI
The utility of annotated image datasets transcends simple data collection. Here are several compelling reasons why they are essential:
1. Facilitating Machine Learning and Deep Learning
In the realm of machine learning, algorithms learn patterns from data. Annotated image datasets provide the structured information required for models to discern features and make predictions. Without these datasets, training AI models would be akin to navigating a labyrinth without a map.
2. Improving Accuracy and Efficiency
High-quality annotations help improve the accuracy of AI systems. For instance, a well-annotated dataset allows algorithms to understand subtle differences between similar objects, enhancing their precision in classification tasks. This translates to improved performance in real-world applications, such as autonomous vehicles, medical imaging analysis, and facial recognition technology.
3. Enabling Transfer Learning
Transfer learning, a technique where a model developed for a specific task is reused as the starting point for a model on a second task, relies heavily on annotated datasets. By leveraging pre-trained models on large annotated image datasets, companies can drastically reduce the time and resources required to achieve accurate results in their specific applications.
4. Addressing Bias and Fairness
Annotated datasets can also help in identifying and mitigating bias in AI models. By ensuring diverse and representative annotations, data scientists can reduce the risk of deploying biased AI solutions, which is crucial for ethical AI practices.
How Data Annotation Tools Enhance the Creation of Annotated Image Datasets
The effectiveness of annotated image datasets is largely dependent on the tools and platforms used for data annotation. Here’s how data annotation tools enhance this process:
1. Streamlined Annotation Processes
Data annotation tools simplify the annotation process, allowing teams to collaborate and annotate images swiftly. This streamlining leads to faster turnaround times and reduced bottlenecks, enabling organizations to harness the benefits of annotated datasets more quickly.
2. Quality Control Mechanisms
Advanced annotation platforms often feature quality control mechanisms that ensure the consistency and accuracy of annotations. This includes features like review systems, automated checks, and user-friendly interfaces for annotators, which collectively contribute to higher-quality annotated image datasets.
3. Scalability
As projects expand, the need for larger datasets becomes apparent. Scalable data annotation platforms allow businesses to annotate large volumes of images efficiently. This scalability is essential for training deep learning models that require extensive datasets to perform adequately.
4. Integration with AI Workflows
Modern data annotation tools often integrate seamlessly with machine learning frameworks and deployment pipelines. This integration facilitates a more cohesive workflow, as teams can move from data annotation to model training and eventually to deployment with minimal friction.
Examples of Applications Utilizing Annotated Image Datasets
Annotated image datasets boast a vast range of applications across various industries. Here are some notable examples:
- Healthcare: AI systems can analyze medical images for abnormalities, relying on annotated datasets of X-rays, MRIs, and CT scans.
- Autonomous Vehicles: Self-driving cars utilize annotated datasets to understand and react to their environments, enabling safer navigation.
- Retail: Computer vision technology in retail uses annotated images to enhance customer experiences, streamline inventory management, and improve loss prevention.
- Security: Facial recognition systems depend on precise annotated datasets to identify individuals accurately in surveillance footage.
The Future of Annotated Image Datasets
The journey of annotated image datasets is far from over. As AI continues to advance, we can expect several emerging trends:
1. Evolution of Annotation Techniques
New methods of annotation, such as automated or semi-automated annotation tools, will likely gain traction. These techniques can significantly speed up the data preparation process, making it easier to create large annotated datasets.
2. Expansion of Datasets
As industries recognize the potential of AI, the demand for comprehensive annotated image datasets will increase. This growth will likely will lead to the creation of more specialized datasets tailored to specific applications or sectors.
3. Enhancing Data Diversity
To combat biases in AI systems, a greater emphasis on diverse and inclusive datasets will emerge. This shift is crucial for ensuring fairness and accuracy in AI applications across different demographics and contexts.
4. Increased Collaboration Across Fields
Cross-industry collaboration will likely arise as organizations share annotated datasets to accelerate research and development. Such collaboration could enhance the collective understanding of various fields while helping to uphold data privacy and security standards.
Conclusion
In summary, annotated image datasets serve as the backbone of successful AI applications. Their importance cannot be overstated, as they enable machine learning systems to learn, grow, and adapt in an increasingly complex world. Utilizing advanced data annotation tools and platforms, such as those offered by keylabs.ai, organizations can harness the power of annotated datasets to drive innovation and transformation across diverse industries. As we continue to explore the potential of AI, the focus on quality annotated datasets will remain a cornerstone of progress, shaping the technologies of tomorrow.